
Surveys
The major purpose of surveys is to describe the characteristics of a population. All kinds of people in all kind of professions are used surveys to gain information about their target populations. There are two main types of surveys; a cross-sectional survey and a longitudinal survey.
Types of surveys
- A cross-sectional survey collects information from a sample at one point in time. When an entire population is surveyed, it's call census. Cross-sectional surveys are useful in assessing practices, attitudes, knowledge, and beliefs of a population in relation to a particular related event.
- A longitudinal survey collects information at different points in time in order to study changes over time. Three longitudinal designs are commonly employed in survey research: trend studies, cohort studies, and panel studies.
In a trend study, different samples from the same population at different points in time. If random selection were used to obtain the samples. These could be considered representative of the population.
2) Cohort studies
A cohort study samples a particular population whose numbers do not change over the course of the survey. A cohort sample has experiences some type of event in a selected time period, and studying them at intervals through time.
3) Panel studies
A panel study is a longitudinal study of a cohort people (same individual) with multiple measures over time. The various data collections are often called waves. Panel studies with several waves are the best quasi-experimental design for investigating the causes and consequences of changes with high internal validity. Moreover, most of big panel studies utilize population probability samples that permit generalization to the target population and provide for external validity. However, big panel studies tend to be very expensive and difficult to conduct.

Advantages and disadvantages of surveys
Advantages
1. Convenient of collecting data, surveys can be administered to the participants through a variety of ways, eg. mail, e-mail, online, mobile, face-to-face, and can be administered in remote area.
2. Little or no observer subjectivity.
3. Participants can take their time to complete the question. Online and e-mail surveys allow respondents to maintain their anonymity.
4. Due to the large number of people who answer surveys, good statistic significant results can be find easier. Moreover, with survey software, advance statistical technique can be utilized.
5. Assuming well-constructed question and study design, survey method is potential to produce reliable results.
6. The representativeness of the respondents makes the surveys potential for generalization.
7. Cost effective, but cost depends on survey method.
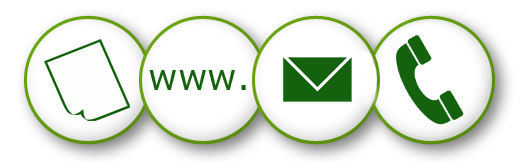
Disadvantages
1. The questions may not appropriate for all of participants. These make differences in understanding and interpretation. The answer of participants may not be precisely answered.
2. Respondents may not feel comfortable providing answers that present themselves in an unfavorable manner. Dishonesty can be an issue.
3. If questions are not required, the respondents will ignore to answer some questions.
4. Open-ended questions are difficult to analyze because of too much of varied opinion.
5. Low response rate.
To read more how to identifying relevant guidance for survey research and evidence on the quality of reporting survey, please go to this link http://journals.plos.org/plosmedicine/article?id=10.1371/journal.pmed.1001069